by Corynne McSherry
Electronic Frontier Foundation
Reprinted under Creative Commons License
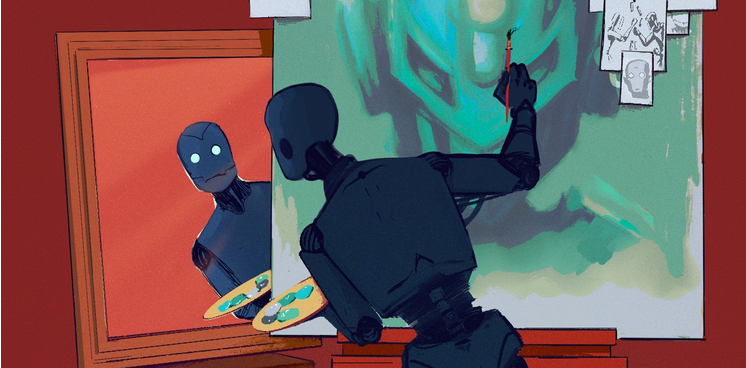
Anxiety about generative AI is growing almost as fast as the use of the technology itself, fueled by dramatic rhetoric from prominent figures in tech, entertainment, and national security. Something, they suggest, must be done to stave off any number of catastrophes, from the death of the artist to the birth of new robot overlords.
Given the often hyperbolic tone, it might be tempting (and correct) to dismiss much of this as the usual moral panic new technologies provoke, or self-interested hype. But there are legitimate concerns in the mix, too, that may require some rules of the road. If so, policymakers should answer some important questions before crafting or passing on those rules. As always, the devil is in the details, and EFF is here to help you sort through them to identify solid strategies and potential collateral damage.
First, policymakers should be asking whether the legislation is both necessary and properly focused. Generative AI is a category of general-purpose tools with many valuable uses. For every image that displaces a potential low-dollar commission for a working artist, there are countless more that don't displace anyone's living—images created by people expressing themselves or adding art to projects that would simply not have been illustrated. Remember: the major impact of automated translation technology wasn't displacing translators—it was the creation of free and simple ways to read tweets and webpages in other languages when a person would otherwise not know what was being said.
But a lot of the rhetoric we are hearing ignores those benefits and focuses solely on potentially harmful uses, as if the tool itself were the problem (rather than how people use it). The ironic result: a missed opportunity to pass and enforce laws that can actually address those harms.
For example, if policymakers are worried about privacy violations stemming from the collection and use of images and personal information in generative AI, a focus on the use rather than the tool could lead to a broader law: real and comprehensive privacy legislation that covers all corporate surveillance and data use. Ideally, that law would both limit the privacy harms of AI (generative and other forms) and be flexible enough to stay abreast of new technological developments.
But sometimes we don't need a new law— we just need to do a better job with the ones we already have. If lawmakers are worried about misinformation, for example, they might start by reviewing (and, where necessary, strengthening) resources for enforcement of existing laws on fraud and defamation. It helps that courts have spent decades assessing those legal protections and balancing them against countervailing interests (such as free expression); it makes little sense to relitigate those issues for a specific technology. And where existing regulations are truly ineffective in other contexts, proponents must explain why they will be more effective against misuses of generative AI.
Second, are the harms the proposal is supposed to alleviate documented or still speculative? For example, for years lawmakers (and others) have raised alarms about the mental health effects of social media use. But there's little research to prove it, which makes it difficult to tailor a regulatory response. In other areas, such as the encryption debate, we're seen how law enforcement's hypothetical or unproven concerns, raised under the flag of "online safety," are used to justify undermining essential protections for online expression and privacy. To create evidence-based laws, policymakers must review the research, talk to experts and civil society (not just CEOs), and watch out for AI snake oil or magical thinking.
Third and relatedly, to what extent does a new proposal embrace speculative harms at the expense of addressing practical and well documented existing harms? Thoughtful researchers and civil society groups have been sounding the alarm for over a decade about the risks of predictive policing, government use of facial recognition systems, and biased decision-making in housing, hiring, and government benefits. We should not let hyperbole and headlines about the future of generative AI distract us from addressing the damage being done today. There is much that can and should be done to ensure transparency, empower communities to reject or select technologies, engage in impact assessment, and create accountability and remedies for those already suffering from those harms. People serious about ensuring that generative AI serves humanity already have a full agenda that shouldn't be swept aside because of rich-guy hype.
Fourth, will the regulation reinforce existing power dynamics and oligopolies? When Big Tech asks to be regulated, we must ask if those regulations might effectively cement Big Tech's own power. For example, we've seen multiple proposals that would allow regulators to review and license AI models, programs, and services. Government licensing is the kind of burden that big players can easily meet; smaller competitors and nonprofits, not so much. Indeed, it could be prohibitive for independent open-source developers. We should not assume that the people who built us this world can fix the problems they helped create; if we want AI models that don't replicate existing social and political biases, we need to make enough space for new players to build them.
Fifth, is the proposed regulation flexible enough to adapt to a rapidly evolving technology? Technology often changes much faster than the law, and those changes can be difficult to predict. As a result, today's sensible-seeming rule can easily become tomorrow's security weak point. What is worse, locking in specific technologies can prevent new and innovative technologies from flourishing and, again, give today's winners the ability to prevent future competitors from offering us a better tool or product.
Sixth, will the law actually alleviate the harm it targets? This question gets overlooked far too often. For example, there have been several proposals to require generative AI users and developers to "watermark" the works they produce. Assuming this is technically possible (it might be harder to do this for music, say, than images), history suggests it won't be very effective against the uses we might worry about the most. "Advisory" watermarking by default, such as DALL-E's automatic insertion of a few colored squares in the corner of an image, can help indicate it was AI-generated, so the person who shares it doesn't unintentionally deceive. But those watermarks can easily be cropped out by the more sophisticated fraudsters we might really want to deter. And "adversarial" watermarking, whereby the AI model generates a watermark that is so deeply embedded in the output that it cannot be removed, has almost always been defeated in practice. In short, watermarking can have some benefits but it's inevitably a cat and mouse game. If we're aiming at serious harms by motivated people, we need strategies that work.
Finally, how does it affect other public interests (beyond the competition issues raised above)? For example, there's a lot of rhetoric around the risks of allowing open-source AI development compared to closed systems where a central authority can control what can and cannot be done by users. We've seen this movie before— open systems are often attacked with this claim, especially by those who benefit from a closed world. Even taking the concern at face value, though, it's hard to see how the government can regulate use and development without restricting freedom of expression and the right to access new information and art. In the U.S., courts have long recognized that code is speech, and policing its development may run afoul of the First Amendment. More generally, just as we would not tolerate a law allowing the government to control access to and use of printing presses, we should worry about giving any central authority the power to control access to generative AI tools and, presumably, decide in advance what kinds of expression those tools can be allowed to generate. Moreover, placing controls on open-source development in some countries may just ensure that developers in other countries have better opportunities to learn and innovate.
Other proposals designed to ensure remuneration for creators whose works are included in training data, such as a new copyright licensing regime, could make socially valuable research based on machine learning and even data mining prohibitively complicated and expensive (assuming such a regime was even administratively possible given the billions of works that might be used and the difficulty of tracking those uses). We have great sympathy for creators who struggle to be properly compensated for their work. But we must look for ways to ensure fair pay that don't limit the potential for all of humanity to benefit from valuable secondary uses. The Writers Guild of America-West's contract negotiations with the movie studios offers one model: AI-generated material can't be used to replace a human writer. Specifically, AI-generated material cannot qualify as source material for adaptation in any way, and if a studio wants to use an AI-generated script, there can be no credited author and, therefore, no copyright. In a world where studios jealously guard the rights to their work, that's a major poison pill. Under this proposal, studios must choose between the upfront cost of paying a writer what they are worth, and the backend cost of not holding a copyright in the ultimate work.
These are questions we should ask of most legislation, as part of a thorough impact assessment. But they are particularly pressing here, where policymakers seem eager to legislate quickly, major players are inviting it, and many of those affected aren't at the table. Where there are real risks to the development of generative AI, and real reasons for people in various sectors to feel ill-used and ignored by the developers, it's even more important for lawmakers to get it right.
|